Top 50 DWBI Interview Questions with Answers - Part 2
This article is the continuation of the article "Top 50 DWBI Interview Questions with Answers"
What is snow-flake schema?
This is another logical arrangement of tables in dimensional modeling where a centralized fact table references number of other dimension tables; however, those dimension tables are further normalized into multiple related tables.
Consider a fact table that stores sales quantity for each product and customer on a certain time. Sales quantity will be the measure here and keys from customer, product and time dimension tables will flow into the fact table. Additionally all the products can be further grouped under different product families stored in a different table so that primary key of product family tables also goes into the product table as a foreign key. Such construct will be called a snow-flake schema as product table is further snow-flaked into product family.
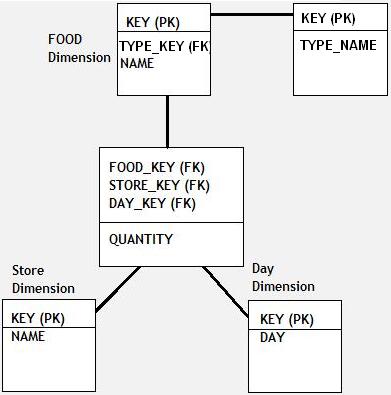
Note: Snow-flake increases degree of normalization in the design.
What are the different types of dimension?
In a data warehouse model, dimension can be of following types,
- Conformed Dimension
- Junk Dimension
- Degenerated Dimension
- Role Playing Dimension
Based on how frequently the data inside a dimension changes, we can further classify dimension as
- Unchanging or static dimension (UCD)
- Slowly changing dimension (SCD)
- Rapidly changing Dimension (RCD)
You may also read, Modeling for various slowly changing dimension and Implementing Rapidly changing dimension to know more about SCD, RCD dimensions etc.
What is a 'Conformed Dimension'?
A conformed dimension is the dimension that is shared across multiple subject area. Consider 'Customer' dimension. Both marketing and sales department may use the same customer dimension table in their reports. Similarly, a 'Time' or 'Date' dimension will be shared by different subject areas. These dimensions are conformed dimension.
Theoretically, two dimensions which are either identical or strict mathematical subsets of one another are said to be conformed.
What is degenerated dimension?
A degenerated dimension is a dimension that is derived from fact table and does not have its own dimension table.
A dimension key, such as transaction number, receipt number, Invoice number etc. does not have any more associated attributes and hence can not be designed as a dimension table.
What is junk dimension?
A junk dimension is a grouping of typically low-cardinality attributes (flags, indicators etc.) so that those can be removed from other tables and can be junked into an abstract dimension table.
These junk dimension attributes might not be related. The only purpose of this table is to store all the combinations of the dimensional attributes which you could not fit into the different dimension tables otherwise. Junk dimensions are often used to implement Rapidly Changing Dimensions in data warehouse.
What is a role-playing dimension?
Dimensions are often reused for multiple applications within the same database with different contextual meaning. For instance, a "Date" dimension can be used for "Date of Sale", as well as "Date of Delivery", or "Date of Hire". This is often referred to as a 'role-playing dimension'
What is SCD?
SCD stands for slowly changing dimension, i.e. the dimensions where data is slowly changing. These can be of many types, e.g. Type 0, Type 1, Type 2, Type 3 and Type 6, although Type 1, 2 and 3 are most common. Read this article to gather in-depth knowledge on various SCD tables.
What is rapidly changing dimension?
This is a dimension where data changes rapidly. Read this article to know how to implement RCD.
Describe different types of slowly changing Dimension (SCD)
Type 0:
A Type 0 dimension is where dimensional changes are not considered. This does not mean that the attributes of the dimension do not change in actual business situation. It just means that, even if the value of the attributes change, history is not kept and the table holds all the previous data.
Type 1:
A type 1 dimension is where history is not maintained and the table always shows the recent data. This effectively means that such dimension table is always updated with recent data whenever there is a change, and because of this update, we lose the previous values.
Type 2:
A type 2 dimension table tracks the historical changes by creating separate rows in the table with different surrogate keys. Consider there is a customer C1 under group G1 first and later on the customer is changed to group G2. Then there will be two separate records in dimension table like below,
Key | Customer | Group | Start Date | End Date |
1 | C1 | G1 | 1st Jan 2000 | 31st Dec 2005 |
2 | C1 | G2 | 1st Jan 2006 | NULL |
Note that separate surrogate keys are generated for the two records. NULL end date in the second row denotes that the record is the current record. Also note that, instead of start and end dates, one could also keep version number column (1, 2 … etc.) to denote different versions of the record.
Type 3:
A type 3 dimension stored the history in a separate column instead of separate rows. So unlike a type 2 dimension which is vertically growing, a type 3 dimension is horizontally growing. See the example below,
Key | Customer | Previous Group | Current Group |
1 | C1 | G1 | G2 |
This is only good when you need not store many consecutive histories and when date of change is not required to be stored.
Type 6:
A type 6 dimension is a hybrid of type 1, 2 and 3 (1+2+3) which acts very similar to type 2, but only you add one extra column to denote which record is the current record.
Key | Customer | Group | Start Date | End Date | Current Flag |
1 | C1 | G1 | 1st Jan 2000 | 31st Dec 2005 | N |
2 | C1 | G2 | 1st Jan 2006 | NULL | Y |
What is a mini dimension?
Mini dimensions can be used to handle rapidly changing dimension scenario. If a dimension has a huge number of rapidly changing attributes it is better to separate those attributes in different table called mini dimension. This is done because if the main dimension table is designed as SCD type 2, the table will soon outgrow in size and create performance issues. It is better to segregate the rapidly changing members in different table thereby keeping the main dimension table small and performing.